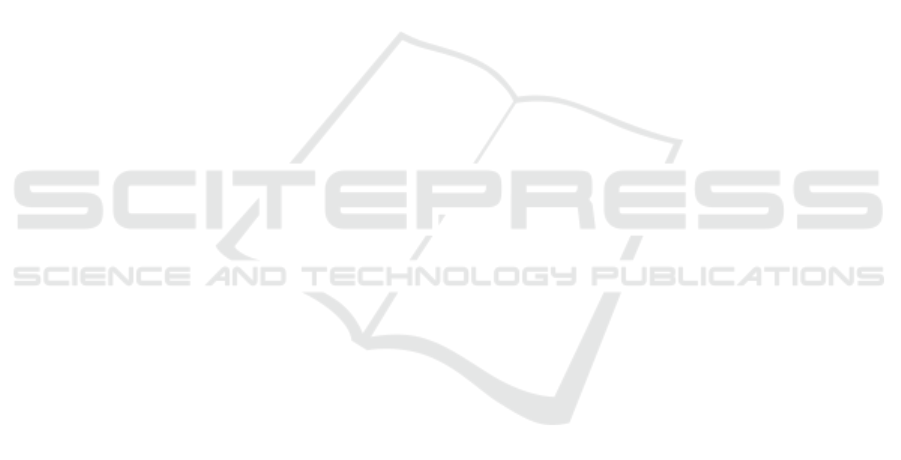
Thoma, G. R. (2009). Annotation and retrieval of clin-
ically relevant images. international journal of medi-
cal informatics, 78(12):e59–e67.
Dhawan, A. P. (2011). Medical image analysis. John Wiley
& Sons.
Dosovitskiy, A., Beyer, L., Kolesnikov, A., Weissenborn,
D., Zhai, X., Unterthiner, T., Dehghani, M., Minderer,
M., Heigold, G., Gelly, S., et al. (2020). An image is
worth 16x16 words: Transformers for image recogni-
tion at scale. arXiv preprint arXiv:2010.11929.
Frome, A., Corrado, G. S., Shlens, J., Bengio, S., Dean,
J., Ranzato, M., and Mikolov, T. (2013). Devise: A
deep visual-semantic embedding model. Advances in
neural information processing systems, 26.
Hasan, M. R., Layode, O., and Rahman, M. (2023).
Concept detection and caption prediction in image-
clefmedical caption 2023 with convolutional neural
networks, vision and text-to-text transfer transform-
ers. In CLEF2023 Working Notes, CEUR Workshop
Proceedings, Thessaloniki, Greece. CEURWS.org.
He, K., Zhang, X., Ren, S., and Sun, J. (2016). Deep resid-
ual learning for image recognition. In Proceedings of
the IEEE conference on computer vision and pattern
recognition, pages 770–778.
Huang, G., Liu, Z., Van Der Maaten, L., and Weinberger,
K. Q. (2017). Densely connected convolutional net-
works. In Proceedings of the IEEE conference on
computer vision and pattern recognition, pages 4700–
4708.
Ionescu, B., M
¨
uller, H., Dr
˘
agulinescu, A. M., Popescu, A.,
Idrissi-Yaghir, A., Garc
´
ıa Seco de Herrera, A., An-
drei, A., Stan, A., Stor
˚
as, A. M., Abacha, A. B., et al.
(2023). Imageclef 2023 highlight: Multimedia re-
trieval in medical, social media and content recom-
mendation applications. In European Conference on
Information Retrieval, pages 557–567. Springer.
Ionescu, B., M
¨
uller, H., P
´
eteri, R., R
¨
uckert, J., Abacha,
A. B., de Herrera, A. G. S., Friedrich, C. M., Bloch,
L., Br
¨
ungel, R., Idrissi-Yaghir, A., et al. (2022).
Overview of the imageclef 2022: Multimedia retrieval
in medical, social media and nature applications.
In International Conference of the Cross-Language
Evaluation Forum for European Languages, pages
541–564. Springer.
Kaliosis, P., Moschovis, G., Charalambakos, F., Pavlopou-
los, J., and Androutsopoulos, I. (2023). Aueb
nlp group at imageclefmedical caption 2023. In
CLEF2023 Working Notes, CEUR Workshop Pro-
ceedings, Thessaloniki, Greece. CEUR-WS.org.
Li, J., Li, D., Xiong, C., and Hoi, S. (2022). Blip:
Bootstrapping language-image pre-training for unified
vision-language understanding and generation. In In-
ternational Conference on Machine Learning, pages
12888–12900. PMLR.
Liu, S. and Deng, W. (2015). Very deep convolutional
neural network based image classification using small
training sample size. In 2015 3rd IAPR Asian confer-
ence on pattern recognition (ACPR), pages 730–734.
IEEE.
Liu, Z., Lin, Y., Cao, Y., Hu, H., Wei, Y., Zhang, Z., Lin,
S., and Guo, B. (2021). Swin transformer: Hierar-
chical vision transformer using shifted windows. In
Proceedings of the IEEE/CVF international confer-
ence on computer vision, pages 10012–10022.
Manzari, O. N., Ahmadabadi, H., Kashiani, H., Shokouhi,
S. B., and Ayatollahi, A. (2023). Medvit: a ro-
bust vision transformer for generalized medical image
classification. Computers in Biology and Medicine,
157:106791.
Mohamed, S. S. N. and Srinivasan, K. (2023). Ssn
mlrg at caption 2023: Automatic concept detection
and caption prediction using conceptnet and vision
transformer. In CLEF2023 Working Notes, CEUR
Workshop Proceedings, Thessaloniki, Greece. CEUR-
WS.org.
Okolo, G. I., Katsigiannis, S., and Ramzan, N. (2022). Ievit:
An enhanced vision transformer architecture for chest
x-ray image classification. Computer Methods and
Programs in Biomedicine, 226:107141.
Pelka, O., Koitka, S., R
¨
uckert, J., Nensa, F., and Friedrich,
C. M. (2018). Radiology objects in context (roco):
a multimodal image dataset. In Intravascular Imag-
ing and Computer Assisted Stenting and Large-Scale
Annotation of Biomedical Data and Expert Label
Synthesis: 7th Joint International Workshop, CVII-
STENT 2018 and Third International Workshop, LA-
BELS 2018, Held in Conjunction with MICCAI 2018,
Granada, Spain, September 16, 2018, Proceedings 3,
pages 180–189. Springer.
Pennington, J., Socher, R., and Manning, C. D. (2014).
Glove: Global vectors for word representation. In
Proceedings of the 2014 conference on empirical
methods in natural language processing (EMNLP),
pages 1532–1543.
Radford, A., Kim, J. W., Hallacy, C., Ramesh, A., Goh, G.,
Agarwal, S., Sastry, G., Askell, A., Mishkin, P., Clark,
J., et al. (2021). Learning transferable visual models
from natural language supervision. In International
conference on machine learning, pages 8748–8763.
PMLR.
Radford, A., Wu, J., Child, R., Luan, D., Amodei, D.,
Sutskever, I., et al. (2019). Language models are un-
supervised multitask learners. OpenAI blog, 1(8):9.
Rahman, M. M., Antani, S. K., Demner-Fushman, D., and
Thoma, G. R. (2015). Biomedical image representa-
tion approach using visualness and spatial information
in a concept feature space for interactive region-of-
interest-based retrieval. Journal of Medical Imaging,
2(4):046502–046502.
Rio-Torto, I., Patr
´
ıcio, C., Montenegro, H., Gonc¸alves, T.,
and Cardoso, J. S. (2023). Detecting concepts and
generating captions from medical images: Contribu-
tions of the vcmi team to imageclefmedical caption
2023. In CLEF2023 Working Notes, Thessaloniki,
Greece. CEUR-WS.org, CEUR Workshop Proceed-
ings.
Ritter, F., Boskamp, T., Homeyer, A., Laue, H., Schwier,
M., Link, F., and Peitgen, H.-O. (2011). Medical im-
age analysis. IEEE pulse, 2(6):60–70.
Sanh, V., Debut, L., Chaumond, J., and Wolf, T. (2019).
Image and Text Feature Based Multimodal Learning for Multi-Label Classification of Radiology Images in Biomedical Literature
685